Recognition of Patterns of Maleficent Objects on Medical Images
Szilvia Nagy
Description
Keywords: medical image; pattern recognition; image processing; colorectal tumor; endoscopy; blood vessels; narrow band imaging
H2020 challenge: Health, demographic change and wellbeing
Knowledge and skills (P: prerequisite; D: desirable, but not necessary): basic knowledge on image taking methods (P); basic knowledge on learning algorithms and soft decision techniques (D); basic image processing (D); basic knowledge on medical imaging (D)
The medical staff is trained to identify maleficent tumours of their specialization field, but an automatic image processing can help their decision, moreover in some cases, like the skin cancers, it could add a tool into the hands of the not specialized persons as well. The non-invasive medical imaging [1][2] contains both the visible light techniques (like endoscopes and microscopes) as well as the higher and lower frequency electromagnetic field based imaging (like X-ray and ultrasound reflection based images). In case of tumours many tell-tale signs can be from the variation of the blood vessel structure around the formula [4-6] to the pattern and colour of the surface of the polyp itself [7-10].
Five INNOSOC students, supervised by two INNOSOC lecturers, will collaborate on answering how ICT can be used in image-based cancer detection. These activities will be conducted as a part of the ERASMUS+ blended mobility and will be finalized during INNOSOC Zagreb 2016 workshop in late April 2016.
Cancer detection is still made by humans, and it will remain so, however, a visual aid can increase effectiveness, and a pre-screening can still be carried out by less qualified personnel and intelligent computer programmes instead of fully trained medical specialists.
The aim of this Case Study is to summarize the technologies used in image-based cancer detection for some types of cancer and compare the applicability of the techniques to the various cases. It is also necessary to determine whether new image processing methods could be used. In most cases the decision about a formula is taken in a crisp, yes or no way, however, many soft decision techniques can also be applied, completed with a learning algorithm, thus it is also necessary to map the applied training algorithms and their efficiency.
Therefore, this Case Study specifically addresses the “Health, demographic change and wellbeing” H2020 challenge.
First, the innovation aspect emerges from comparing multiple types of image processing methods used for various purposes that can lead to a common method or a method applied for one type of problem to be applicable in other problems.
Second, although medical image processing is an international problem, acquiring images has different aspects in different cultures. Additionally, interpreting and communicating the results has various cultural aspects as well.
Third, medical images are processed by IT devices, and their transmission has multiple ICT tasks from coding, compressing to videoconferences about the results.
Questions that need answers
- What are image acquiring methods used in tumour classification?
- Are there specially developed imaging techniques for some aspects of tumour detection (e.g., for recognition of surfaces and interfaces of tumours or for enhancing the visibility of the blood vessels)?
- What are the most lethal cancer types in Europe and in the other continents?
- What is the usual diagnose method for the most lethal tumours?
- What type of image processing methods are used in detection or classification of the most dangerous tumour types?
- What type of soft or crisp decision techniques are used in tumour classification? What types of evolutionary or learning algorithms are applied?
- How the IT classification success rate is related to the human classification success rate or the biopsy results? What tumour types are well classifiable and what are hard to determine? Why (from image processing point of view)?
Case study supervisors
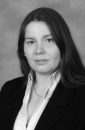
Szilvia Nagy
Lecturer
Case study students
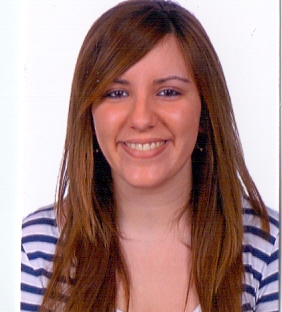
Sandra Yuste Muñoz
INNOSOC 2016 & 2017 Student
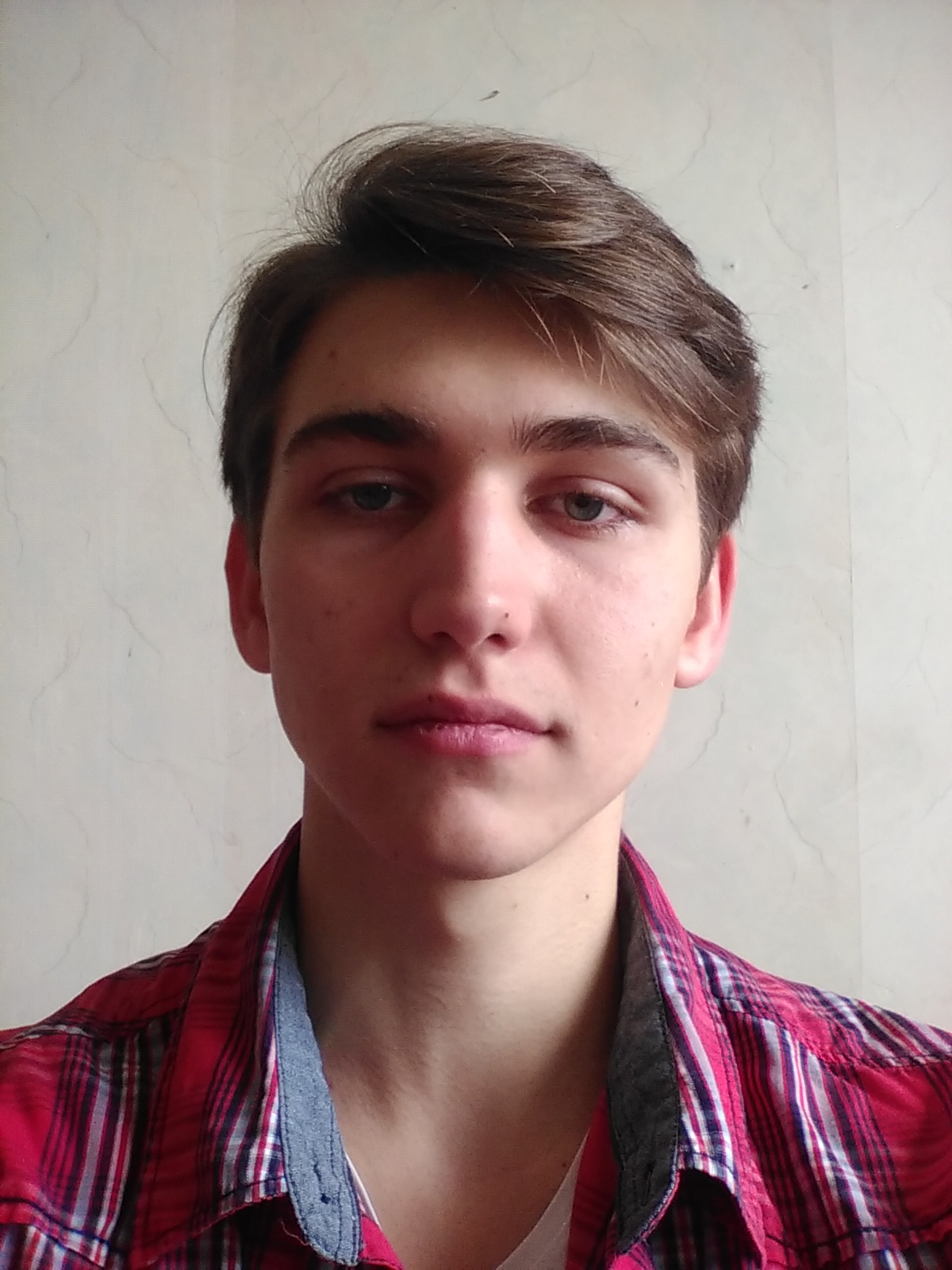
Georgi Nikolov
INNOSOC 2016 Student
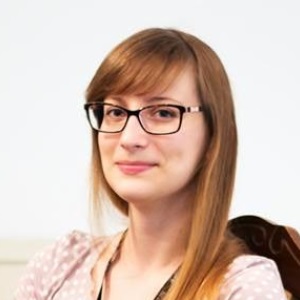
Irena Oršolić
INNOSOC 2016 Student
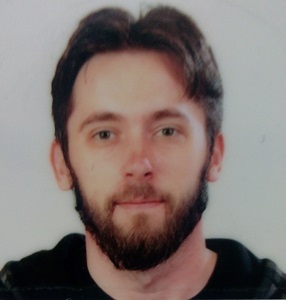